Non-linear Processing (NLP) in echo cancellation refers to operations of removing traces of residual echo in the “send” signal (and, specifically, in the error signal). Figure 1 depicts the NLP block and surrounding blocks and others elements of an echo canceller.
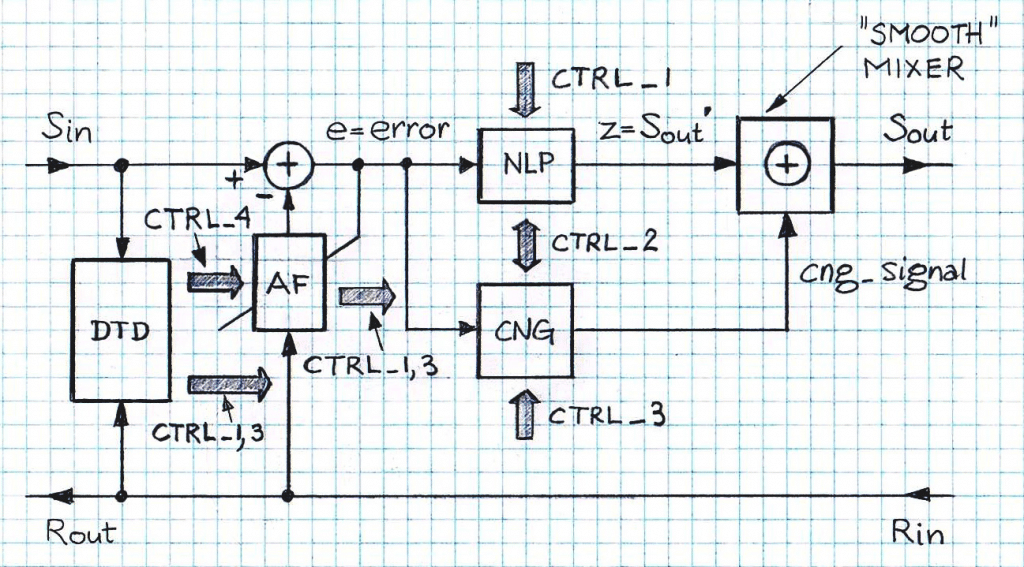
While details of the NLP vary from implementation to implementation, basic operations of the NLP remain largely common for LEC/NEC and AEC cancellers. Conceptually, the fundamental role of the NLP block is to apply large attenuation to the input e (error signal) during Far-End Single-Talk mode so the output z (Sout signal) does not have residual echo components of audible energy. The application of the large attenuation typically is controlled by the Double-Talk Detector only (not shown in Figure 1) although various versions that include control signals coming from other blocks are possible. During Double-Talk mode the NLP should not attenuate signal e at all.
ITU-T G.168-2015 outlines details on testing of the NLP. Also, it provides a description of a “reference Non-linear Processor”. While the referred standard document does not indicate that any practical implementation of an NLP should follow the reference Non-linear Processor, its description helps to communicate appropriate behavior for the NLP block as well as provide details on the design of the NLP tests. The main intent of these tests is to ensure that NLP operations are transparent from the viewpoint of the far-end listener and, at the same time, the traces of residual echo during the Far-End Single-Talk mode are removed from signal e or otherwise mitigated so they are not audible.
In Figure 2, two versions of the “reference Non-linear Processor” basic functionality are depicted. The steady-state input/output nonlinear characteristics, version (a) and version (b), reflect memoryless behavior, which is an idealization of real-life implementations (note that the “steady state” refers to the observation time when all transients and other short-term transitions have died down).
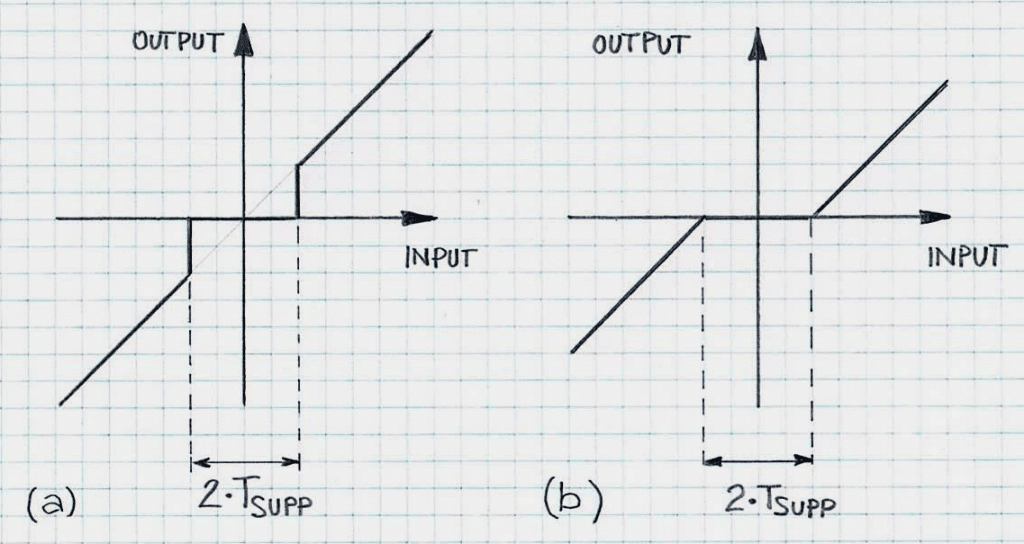
Dynamic performance of the NLP is of critical importance; from the viewpoint of the far-end listener, transitions NLP OFF/NLP ON/NLP OFF, can be effortlessly noticed if they are not smooth and if the near-end speech is nonlinearly distorted (for example, if it is temporarily clipped, or otherwise, cut or corrupted) during these transitions.
In Figure 3 traces of the NLP operate and hangover transitions are shown; they illustrate dynamic transitions from NLP OFF to NLP ON and vice versa.
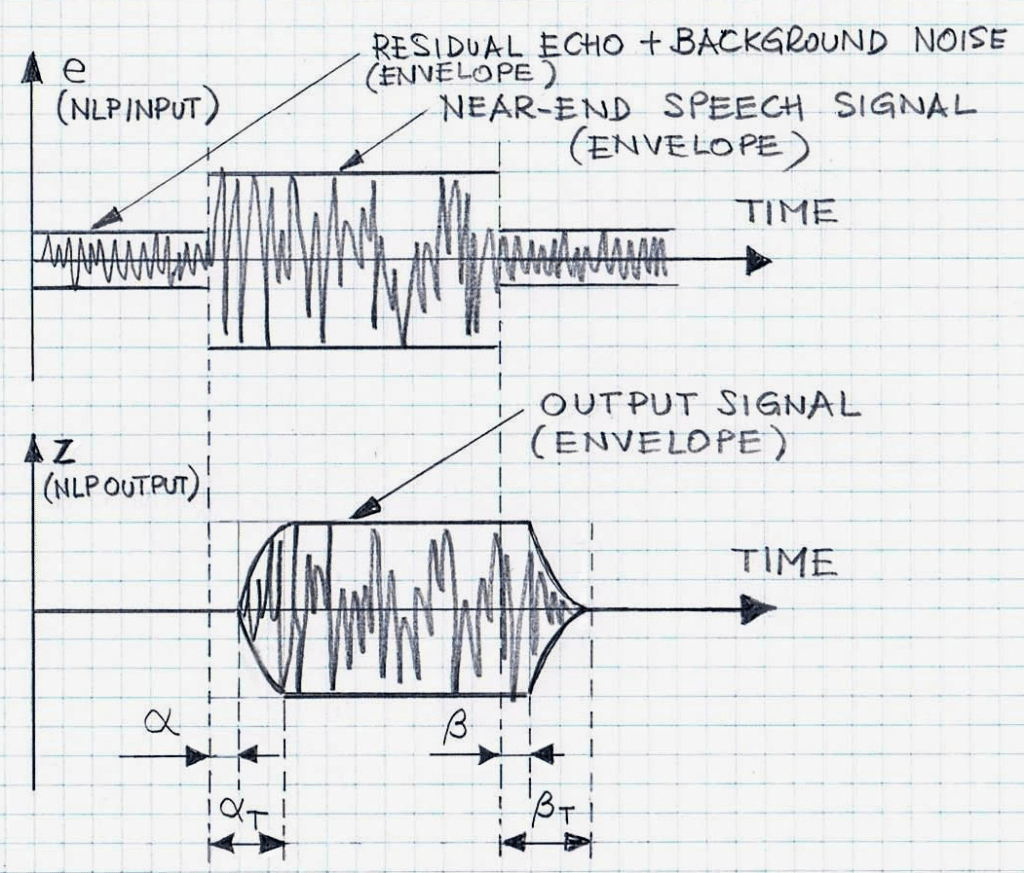
In practical implementations of NLP, specific thresholds, such as TSUPP, are often predefined and in effect at the starting point of echo canceller operation and, as the voice call progresses, they are adjusted according to the actual signal levels and voice call dynamics. These mechanisms are based on proprietary solutions developed for particular applications.
In specific implementations, NLP and CNG (including “smooth” mixing functionality) are considered to be one NLP functional block. Here we treat the NLP block separately for illustration and presentation purposes.
VOCAL Technologies’ echo cancellation solutions are equipped with robust NLP and DTD functionalities. If necessary, these functionalities can be configured to meet specific customer requirements. Please contact us to discuss your echo cancellation application.